AI & MACHINE LEARNING IN RETAIL & DATA ANALYTICS - WHAT'S THE HYPE?
- Caelan McNamara
- Sep 25, 2023
- 5 min read
Updated: Dec 17, 2024
Is it just me or are you also feeling inundated by buzzwords like "Artificial Intelligence (AI)", "Machine Learning (ML)" and “ChatGPT”? There is a lot of hype but it’s not hyperbole to say these technologies are revolutionising retail and data analytics.
Often used interchangeably, AI and ML actually serve different, albeit complementary, purposes and this is why we’re sorting through the hype to explain their distinct roles and delve into their potential. And it'll become clear why data - good, clean, up-to-date data - are crucial to realising the full potential of AI.
Remind Me What Artificial Intelligence Is!
Artificial Intelligence, as a term, encompasses the vast field of computer science dedicated to mimicking human intelligence.
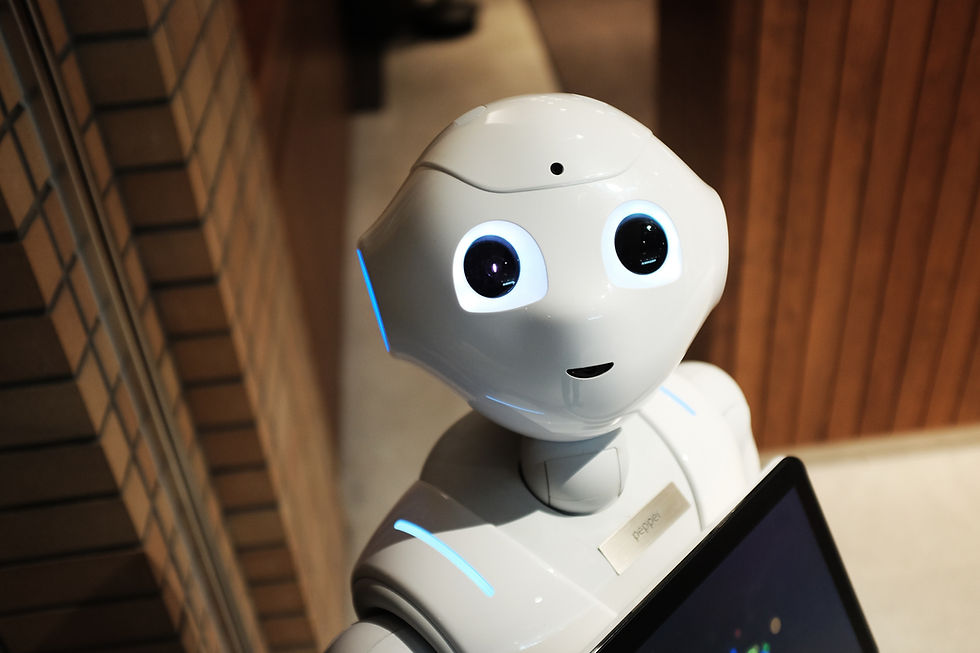
And it's all about the mathematics! Whilst Hollywood may paint AI with a brush of sentient robots and futuristic cities, its core lies in advanced mathematics. Complex algorithms and intricate mathematical models analyse vast swathes of data, diverse in nature, and at great speed, to make predictions and insights. They don’t possess emotions or consciousness; instead, they’re purely results-driven, delivering accurate outcomes based on the data they’ve been fed.
AI ranges from tasks as basic (and boring) as automated rule-following, like customer service chatbots, to more complex feats that involve cognitive problem-solving and decision-making abilities. For example, many retailers and logistics partners use AI-powered robots for storing, picking, and packaging products. And AI algorithms are often used to optimise shipment and last-mile delivery routes.
Simply put, AI is the science of making machines that can think and perform like humans.
So Why is There So Much Hype About AI and Machine Learning at the Moment?
The hype is down to Chat-GPT, a large language model-based chatbot developed by OpenAI, which launched at the end of 2022. It is based on the latest form of AI; generative AI. We’ve all been using traditional AI for years, like when we speak to Alexa, search the web or use a streaming service providing personalised recommendations. Common use cases in retail include:
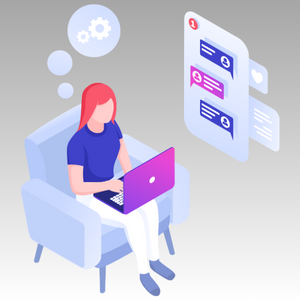
Product discovery and search: AI can improve the accuracy and relevance of product searches
Chatbots: these can enhance customer service and capture data that can be analysed to identify recurring issues
Demand forecasting: AI algorithms can be used to estimate product demand and adjust supply chain processes accordingly.
However, generative AI is an emerging technology that goes beyond traditional AI’s data analysis and prediction because it creates new data from a piece of information it is given. Generative AI is trained on a set of data and learns the underlying patterns to generate new data, which could be text, images, computer code or music. And this is why there is so much media coverage.
One of the most obvious use cases for generative AI is in marketing, particularly content generation. It has the potential to transform how retailers and brands manage marketing content given huge volumes of content (visual and written) can be produced quicker and at less cost. Another potential use case is using synthetic customer profiles, supplementing real data to ensure marketing algorithms are more comprehensive and less biased. Think of it as an evolving component in the AI universe that offers fresh avenues for innovation.
And What's The Difference Between AI and Machine Learning?
Machine Learning, while a cornerstone of AI, narrows the focus to building algorithms that learn from, and make decisions based on data. It may be helpful to imagine AI as the universe with ML as one of its vital galaxies. Deep learning, neural networks, natural language processing and large language models are all solar systems which make up part of the ML galaxy.
Although these terms are often used interchangeably, it can be helpful to remember their differences when analysing their implications for retail analytics.
Using AI and ML in Retail and Data Analytics
Modern retail is much more than its physical and digital stores: they are data generation hubs. Every product, transaction, search, click, product view and customer review feeds this ever-expanding data pool. AI and ML algorithms can rapidly sift through this immense data, decipher patterns, and transform these patterns into actionable strategies. These strategies can lead to better personalisation, increased sales, enhanced customer experience, and more efficient stock management when executed correctly.
The Power of Predictive and Prescriptive Modelling in Retail Operations
With supervised learning, ML can forecast sales trends using pre-defined datasets like historical sales data, helping retailers to plan better, manage supply chains more efficiently, optimise inventory and launch targeted marketing campaigns.

And that's great! But now imagine understanding different customer segments without needing to predefine them. With unsupervised learning, algorithms sift through data without explicit instructions. Techniques like “K-means clustering” do just that; organising customers into segments based on purchasing behaviour, and marketing communications can then become properly personal. Inspired by human brain connections, neural networks can recognise intricate patterns and nuances at speed, making them perfect for systems like product recommendation engines.
Prescriptive analytics goes further by recommending specific actions to achieve desired outcomes. In retail, this could mean using forecast demand to advise on supply chain adjustments or pricing strategies to meet that demand. It is a way for retailers to be proactive, adapting in real-time to market changes.
Powered by Data, AI can Accelerate Digitalisation
Modern retail is powered by data. It’s the same with AI. With high-quality, diverse data, AI algorithms can accelerate digital transformations, enabling businesses to do more with less. Of course, they are only as good as the underlying data! As ever, data quality is the first step toward fully capitalising on these technologies. By focusing on data quality and embracing AI, retailers can drastically improve customer experiences and make better-informed decisions.
Consumer behaviour evolves, meaning yesterday's winning strategies might be obsolete today. Using past behaviours and trends, predictive modelling allows retailers a sneak peek into possible future scenarios. This foresight, plus actionable insights from prescriptive modelling, gives retailers the tools to pivot their strategies and align with market demands.
AI and ML are not mere evolutions but revolutions that promise to make retail businesses more agile, adaptive, and relevant. That’s why there’s so much hype! Are you ready to join the revolution?
If your data is holding you back, talk to us about how to get the most value from your data. Click here to get in touch.
About Datitude
Datitude is transforming how businesses connect, integrate, and unify their data. Our managed data platform and service provide the unifying framework to enable businesses to get what they need from their data, when they need it, in the format needed. A true single source of truth for everything. No silos.
Whilst we made our name in omnichannel working with many retailers and brands over the years, as data experts we provide data solutions to a diverse range of businesses who need better access to their data and valuable intelligence and actionable insight they can trust.
Solutions for retailers and brands include systems integration, a retail & customer data platform, and direct mail optimisation and analysis. Make your data work for you without having to master the underlying technology.